Specialist Data Revisited
In the last article, I went over the issues I have with specialist-based approaches for deriving course fits for players. The quick version is that specialist-based approaches often fail to distinguish which specialist categories are predictive of future outcomes (and to what degree). Today, I’m going to try to replicate the specialist procedure with some metrics of my own and gauge the relative predictive value of some common specialist categories.
First, I should give a short explanation regarding the metric I have chosen to ascertain baseline and condition-specific performance levels. The most commonly used one is strokes gained total. My general issues with strokes gained aside, I think that it’s a decent choice for measuring baseline/specialist performance, since it’s a field-relative metric and thus accounts for differences in course conditions.
I’ll be using something similar, an over/underperformance metric that measures on a scale of -1 to +1 how much a golfer over/underperformed his general expectations in a tournament. It’s also measured relative to the field and has more general rules baked into the expectations (i.e. bombers generally do better on par-72 courses, so all bombers are expected to do better). I think that this metric is a little bit better than SG:T differential, since SG:T doesn’t have those general expectation rules baked in, allowing us to isolate the specialist component a little better.
The Specialist Procedure
To recap the specialist procedure:
- I calculated everyone’s annual average over/underperformance for all courses.
- I calculated their over/underperformance under five different specialist conditions (easy courses, par-70, -71, and -72 courses, and windy conditions).
- I took the difference between average over/underperformance and specialist condition over/underperformance to get condition-specific performance level.
There are a lot of ways to see if prior specialist performance is predictive of future specialist performance: The easiest first pass is to find the year-over-year correlation in specialist performance. If you want a rough idea of what constitutes good year-over-year correlation, I recommend Jake Nichols’ look at different golf stats.
Here are the year-over-year scatter plots for all specialist conditions, 2004-present.
Easy Courses:
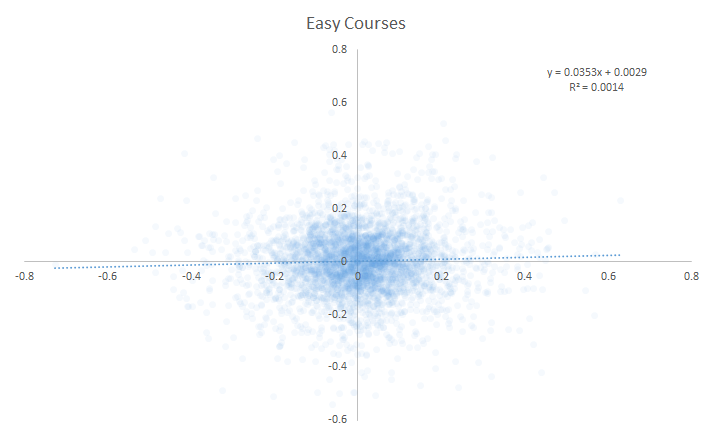
Par-70 Courses:
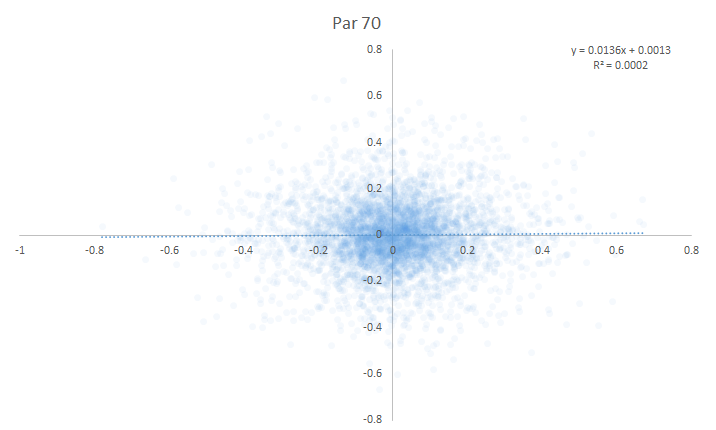
Par-71 Courses:
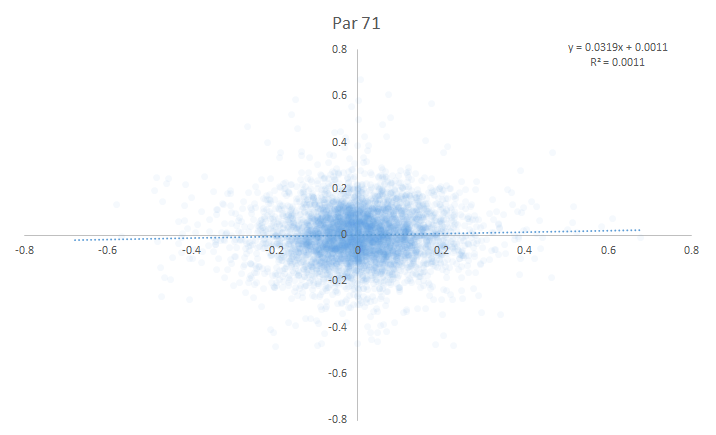
Par-72 Courses:
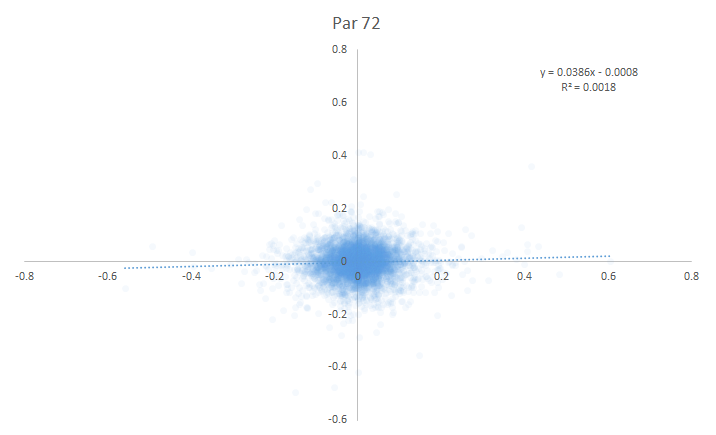
Windy Conditions:
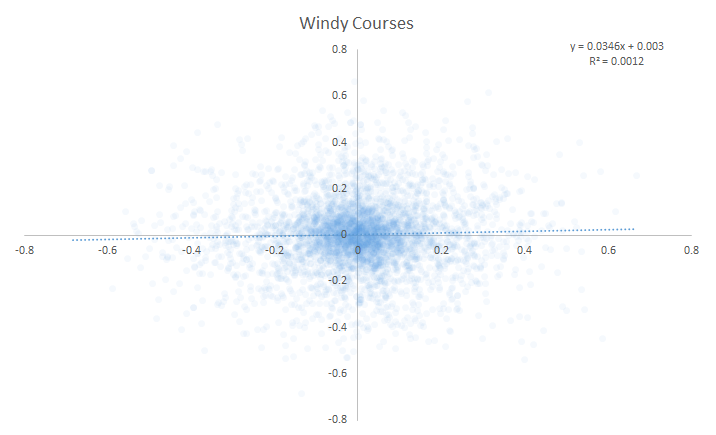
I came into this article hoping that I could at least rank some of these specialist conditions so that we could assess which ones were good relative to one another. Instead . . . I couldn’t find any year-over-year correlation of specialist performance for any of the conditions. On first pass, there’s no evidence that specialist-type metrics are predictive of future outcomes.
This is a first pass, not an exhaustive study. In no particular order, here’s why I think that these results are coming out the way that they are:
- General rules are already doing most of the heavy lifting. I still believe 100 percent that certain playing styles and stats fit certain courses really well and should be accounted for. But why wouldn’t you come to that conclusion organically by weighting those stats directly instead of coming at it from a specialist approach? Specialist-type metrics should always come after applying general rules, since you can calibrate general rules with a much larger sample size and have a better idea of what their weight should be.
- The data is really noisy, so it’s not good at picking up specialist tendencies. I can almost guarantee that this is happening: There is truly someone out there who plays better in wind, but windy conditions are really noisy. Just because I can’t see it in the data, that doesn’t mean that it’s not there — which is exactly my point. If there is a specialist out there, it’s extremely difficult to ascertain from the data. (More on this next week.)
- My internal metric is missing something. “We should be able to see it in the data” is not a catch-all: Different people can see different things, depending on their approaches. I could very well be missing something, and I’m open to refining my approach. I would, though, like to see some affirming evidence as a counter-argument that specialist-type performance is predictive. I’ve seen none to date.
Specialist Metrics Probably Aren’t Very Useful
After all of that, my hunch is still that specialist metrics aren’t all that useful based on my experience trying to fit other individual tendencies to data. It’s hard enough to get good general rules on how to weight things. When you try to apply them at an individual level, there’s just an overwhelming amount of noise that stops you from finding individually predictive stuff.
Somewhat ironically, the only individualized metric I’ve found that works well is course history, which is apparently an open debate. Hopefully I’ll be able to settle that one down the road. But as far as the specialist approach, I was skeptical of its usefulness before doing a data dive, and I’m even more skeptical after.